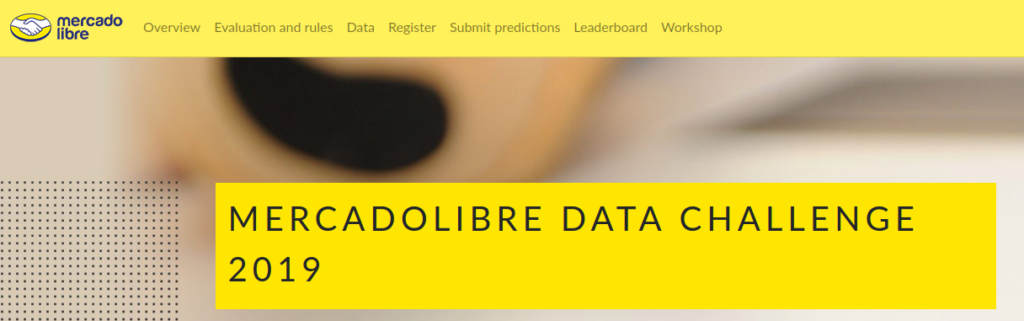
The MercadoLibre Data Challenge 2019 was a great competition Kaggle’s style with an awsome prize consisting on tickets (and accomodation & air tickets) to Khipu Latin American conference on Artificial Intelligence.
I gave it a try implementing several ideas I already had in my head. First I tried a standard BERT embeddings classifier which proved harder to implement in Keras with all the current changes that have been going on with Tensorflow 2.0. Next I tried a Multi Layer Perceptron (MLP) fed with fixed BERT precalculated sentence embeddings. These two approaches didn’t traveled too far but may be interesting starting points to try in the future.
Finally a simpler model gave me the best results I could get, with a Balanced Accuracy Score of 0.87, which put me in the 45st place in the leaderboard. The model uses a Tensorflow Hub Keras layer fine tunned from Neural Probabilistic Language Model described here, followed by a MLP, trained with Adam and Sparse Categorical Crossentropy loss. Check the Github repository for details and code.
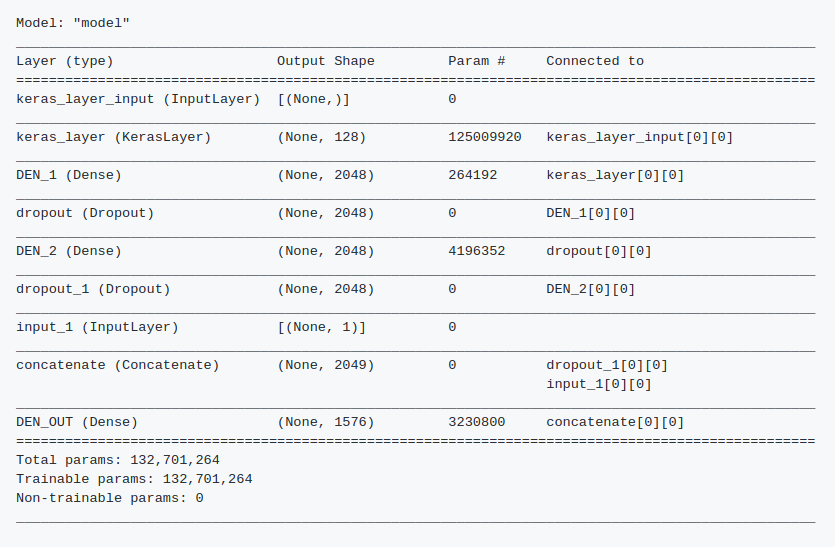
Some interesting findings:
- The language model that I used was spanish based but performed almost equally well in portuguese (nnlm-es-128dim-with-normalization)
- I got slightly better results by training the same model separetly on both languages and merging the results. But a single model trained on the whole dataset perfomed almost as well
The kind guys at MercadoLibre did a kickstart workshop and gave away a model that uses ULMFiT and reaches also 0.87. I think it’s very interesting that my model, using a different approach reaches a very similar result, and that’s why I’m pretty happy with it despite being very far from the first places!